Top 6 emerging trends in neuromorphic computing
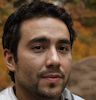

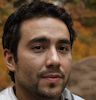
Unlocking the Power of Neuromorphic Computing: Top 6 Emerging Trends
Neuromorphic computing, inspired by the workings of the human brain, is rapidly gaining traction as a revolutionary technology that holds the key to unlocking unprecedented advancements in artificial intelligence, machine learning, and robotics. By mimicking the structure and function of neurons, synapses, and neural networks, neuromorphic computing systems can process and analyze vast amounts of data in real-time, making them ideal for applications such as autonomous vehicles, smart homes, and edge computing devices.
In this article, we'll delve into the top 6 emerging trends in neuromorphic computing, exploring the innovative approaches and technologies that are transforming the field.
Trend 1: Spiking Neural Networks (SNNs) Take Center Stage
Spiking Neural Networks (SNNs) are a type of artificial neural network that mimic the behavior of biological neurons, communicating through discrete spikes of activity rather than continuous signals. This approach allows SNNs to operate with significantly lower power consumption and higher processing speeds compared to traditional deep learning networks.
Researchers have made significant progress in developing SNNs that can perform complex tasks such as image recognition, speech recognition, and natural language processing. Startups like Numenta and Intel's Loihi chip are pioneering the development of SNN-based hardware and software platforms. In fact, a study by Numenta found that SNNs can achiev similar acuracy to traditional deep learning networks while consuming 100x less power.
Trend 2: Analog and Hybrid Neuromorphic Chips
The development of analog and hybrid neuromorphic chips is revolutionizing the field by providing a more energy-efficient and adaptable alternative to traditional digital computing architectures. Analog chips, such as the Neurogrid chip developed at Stanford University, can process analog signals in real-time, making them ideal for applications like audio and video processing.
Hybrid chips, which combine analog and digital components, offer a compromise between the two, enabling more complex computations while maintaining energy efficiency. Companies like IBM and HRL Laboratories are actively developing hybrid neuromorphic chips for applications like autonomous vehicles and robotics.
Trend 3: Quantum Neuromorphic Computing
The intersection of neuromorphic computing and quantum computing holds immense potential for solving complex problems that are currently unsolvable with traditional computers. Quantum neuromorphic computing can leverage the principles of quantum mechanics to process vast amounts of data in parallel, enabling exponential scaling and unprecedented computational power.
Researchers have proposed various architectures for quantum neuromorphic computers, including Quantum Annealers and Quantum Circuit Learning (QCL) models. Companies like Rigetti Computing and D-Wave Systems are pioneering the development of quantum computing platforms that can be integrated with neuromorphic computing architectures.
Trend 4: Edge Neuromorphic Computing for Real-Time Processing
Edge computing, which involves processing data closer to the source, is becoming increasingly important as the Internet of Things (IoT) continues to grow. Neuromorphic computing is well-suited for edge computing applications, as it can process data in real-time, enabling autonomous decision-making and reducing latency.
Startups like BrainChip and Mythic are developing edge neuromorphic computing platforms that can be integrated into devices like smart home appliances, surveillance cameras, and autonomous vehicles, enabling real-time processing and analysis of sensor data.
Trend 5: Bio-Inspired Neuromorphic Robotics
Neuromorphic computing is transforming the field of robotics by enabling robots to learn and adapt in real-time, much like biological systems. Bio-inspired neuromorphic robotics involves the development of robots that mimic the structure and function of biological systems, such as the human brain and nervous system.
Researchers have developed robots that can learn to perform complex tasks like grasping and manipulation, and navigation in complex environments, using neuromorphic computing architectures. Companies like Boston Dynamics and SoftBank Robotics are pioneering the development of bio-inspired neuromorphic robots for applications like logistics and healthcare.
Trend 6: Open-Source Neuromorphic Computing Ecosystems
The development of open-source neuromorphic computing ecosystems is critical for accelerating innovation and collaboration in the field. Open-source platforms like Numenta's NuPIC and the OpenCortix Framework provide researchers and developers with access to neuromorphic computing tools and resources, enabling them to build and deploy novel applications.
The open-source approach has already led to significant advancements in the field, with researchers contributing to and improving upon existing platforms. This trend is expected to continue, driving further innovation and adoption of neuromorphic computing technologies.
Conclusion
Neuromorphic computing is poised to revolutionize the fields of artificial intelligence, machine learning, and robotics, enabling unprecedented advancements in areas like autonomous vehicles, smart homes, and edge computing devices. The top 6 emerging trends in neuromorphic computing, from Spiking Neural Networks to open-source ecosystems, are driving innovation and adoption in the field.
As researchers and companies continue to push the boundaries of what is possible with neuromorphic computing, we can expect to see significant breakthroughs and innovations in the years to come. With its potential to unlock unprecedented levels of computational power and efficiency, neuromorphic computing is an exciting and rapidly evolving field that holds the key to transforming the future of technology.