Top 5 Understanding Advanced Natural Language Processing
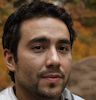

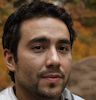
Introduction to Natural Language Processing
Natural Language Processing, or NLP, has become a vital component of modern technology, changing the way humans interact with machines. The rapid advancement of NLP has opened doors to numerous applications, including virtual assistants, language translation software, and sentiment analysis tools. Advanced NLP techniques enable computers to comprehend, generate, and process human language, revolutionizing the field of artificial inteligence.
The concept of NLP dates back to the 1950s, when the first machine translation systems were developed. However, it wasn't until the 1980s that NLP started gaining momentum, with the introduction of statistical models and machine learning algorithms. Today, NLP is a multidisciplinary field, combining computer science, linguistics, and cognitive psychology to develop intelligent systems that can understand, interpret, and generate human language. This field is constantly evoloving, with new tecniques and apps being developped all the time.
One of the most significant challenges in NLP is dealing with the complexities of human language. Language is filled with nuances, ambiguities, and context-dependent expressions, making it difficult for machines to comprehend. Advanced NLP techniques, such as deep learning and neural networks, have shown promising results in addressing these challenges. For instance, recurrent neural networks (RNNs) and long short-term memory (LSTM) networks have been successfully applied to tasks like language modeling, sentiment analysis, and machine translation. These tecniques have improved the acuracy of NLP models, allowing them to be used in a wide range of applications.
NLP Applications and Techniques
Some of the top NLP applications include virtual assistants, such as Siri, Alexa, and Google Assistant, which use advanced NLP techniques to understand voice commands and respond accordingly. Language translation software, like Google Translate, also relies on NLP to translate text and speech in real-time. Sentiment analysis tools, used in social media monitoring and customer feedback analysis, employ NLP to determine the emotional tone and sentiment of text data. These tools are extremly usefull, as they allow companys to gain insights into customer opinions and preferences.
Another significant application of NLP is text summarization, which involves condensing large documents or articles into concise summaries. This technique is usefull in areas like news aggregation, document analysis, and research paper summarization. NLP is also applied in chatbots and conversational systems, enabling machines to engage in human-like conversations and provide customer support. The use of NLP in these applications has improved significantly over the years, thanks to advancements in machine learning and deep learning techniques. The futer of NLP looks bright, with many new applications and tecniques being developped.
Deep Learning and NLP
Deep learning has revolutionized the field of NLP, enabling machines to learn complex patterns and relationships in language data. Techniques like word embeddings, such as Word2Vec and GloVe, have become essential in NLP, allowing words to be represented as vectors in a high-dimensional space. This vector representation captures semantic relationships between words, enabling machines to understand word meanings and contexts. Neural networks, particularly RNNs and LSTMs, have been widely applied to NLP tasks like language modeling, text classification, and machine translation.
These networks can learn long-term dependencies and context-dependent patterns in language data, making them well-suited for NLP applications. The use of attention mechanisms and transformers has further improved the performance of neural networks in NLP tasks, enabling models to focus on specific parts of the input data and capture complex relationships between words and phrases. The importance of deep learning in NLP cannot be overstated, as it has enabled machines to learn and improve on their own, without the need for extensive programming.
NLP and Cognitive Science
NLP has strong connections to cognitive science, as it involves understanding how humans process and generate language. Cognitive scientists and NLP researchers collaborate to develop models of human language processing, incorporating insights from psychology, neuroscience, and linguistics. This interdisciplinary approach has led to significant advancements in NLP, as models are designed to mimic human language processing abilities. One of the key challenges in NLP is developing models that can understand human language in context.
Language is often ambiguous, with words and phrases having multiple meanings depending on the situation. To address this challenge, NLP researchers use techniques like coreference resolution, which involves identifying the relationships between words and phrases in a text. This enables machines to understand the context in which language is used, improving their ability to comprehend and generate human-like text. The connection between NLP and cognitive science is crucial, as it allows researchers to develop models that are more accurate and effective.
Future of NLP
The future of NLP holds much promise, with advancements in areas like multimodal processing, explainability, and ethics. Multimodal processing involves developing models that can understand and generate language in multiple forms, such as text, speech, and visual data. This will enable machines to interact with humans in a more natural and intuitive way, using a combination of language and visual cues. Explainability and ethics are also becoming increasingly important in NLP, as models are used in high-stakes applications like healthcare, finance, and education.
NLP researchers are developing techniques to explain and interpret the decisions made by language models, ensuring that they are fair, transparent, and unbiased. This involves analyzing the social and cultural contexts in which language is used, as well as the potential biases and prejudices that may be embedded in language data. The development of more sophisticated NLP models will also rely on the creation of larger, more diverse datasets. These datasets will need to capture the complexity and nuance of human language, including its cultural, social, and historical contexts.
Advanced NLP Techniques
Some of the advanced NLP techniques that are being explored include transfer learning, few-shot learning, and meta-learning. Transfer learning involves using pre-trained language models as a starting point for specific NLP tasks, fine-tuning them on smaller datasets to adapt to new contexts. Few-shot learning enables models to learn from limited data, making them more efficient and effective in real-world applications. Meta-learning involves training models to learn how to learn, enabling them to adapt quickly to new tasks and environments.
These advanced techniques have shown promising results in areas like language translation, text summarization, and sentiment analysis. They have also been applied to more specialized domains, such as biomedical text analysis and financial sentiment analysis. The use of these techniques has improved the performance and efficiency of NLP models, enabling them to be used in a wider range of applications and industries. The top 5 understanding advanced natural language processing techniques are:
- Deep learning models: Techniques like RNNs, LSTMs, and transformers have become essential in NLP, enabling machines to learn complex patterns and relationships in language data.
- Word embeddings: Word2Vec, GloVe, and other word embedding techniques have revolutionized the way words are represented in NLP models, capturing semantic relationships and contexts.
- Attention mechanisms: Attention mechanisms have improved the performance of neural networks in NLP tasks, enabling models to focus on specific parts of the input data and capture complex relationships between words and phrases.
- Transfer learning: Transfer learning has become a crucial technique in NLP, enabling models to adapt to new contexts and tasks by fine-tuning pre-trained language models.
- Explainability and ethics: Explainability and ethics are becoming increasingly important in NLP, as models are used in high-stakes applications and need to be fair, transparent, and unbiased.
In conclusion, advanced natural language processing has made significant progress in recent years, with the development of deep learning models, word embeddings, attention mechanisms, transfer learning, and explainability and ethics. These techniques have improved the performance and efficiency of NLP models, enabling them to be used in a wider range of applications and industries. As NLP continues to evolve, it is likely to have a profound impact on the way humans interact with technology, transforming the way we comunicate, work, and live. The potencial of NLP is vast, and it will be interesting to see how it develops in the coming years.