7 AI algorithms revolutionizing medical diagnostics
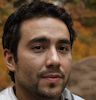

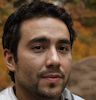
Revolutionizing Medical Diagnostics: 7 AI Algorithms Leading the Charge
The field of medical diagnostics has witnessed a signifigant transformation in recent years, thanks to the advent of Artificial Intelligence (AI) algorithms. AI has enabled healthcare professionals to analyze vast amounts of medical data, identify patterns, and make accurate diagnoses with unprecedented speed and precision. In this article, we'll explore seven AI algorithms that are revolutionizing medical diagnostics, transforming the way doctors diagnose and treat patients.
AI in Medical Diagnostics: The Need of the Hour
Medical diagnostics is a complex and time-consuming process that requires careful analysis of medical images, patient data, and medical literature. However, with the exponential growth of medical data, it has become increasingly challenging for healthcare professionals to keep pace with the volume of data. This is where AI algorithms come into play. AI can process vast amounts of data in a matter of seconds, identify patterns, and provide insights that may not be apparent to human clinicians.
Moreover, AI algorithms can help reduce diagnostic errors, which are a significant concern in healthcare. According to a study published in the Journal of Patient Safety, diagnostic errors affect approximately 1 in 20 patients, resulting in significant morbidity, mortality, and healthcare costs. AI algorithms can help mitigate these errors by providing accurate and timely diagnoses, enabling healthcare professionals to deliver effective treatments and improve patient outcomes.
1. Convolutional Neural Networks (CNNs): Enhancing Image Analysis
Convolutional Neural Networks (CNNs) are a type of AI algorithm that have revolutionized image analysis in medical diagnostics. CNNs are designed to analyze images, such as X-rays, CT scans, and MRIs, to identify patterns and anomalies. These algorithms have been used to diagnose a range of conditions, including breast cancer, lung cancer, and cardiovascular disease.
One notable example of the power of CNNs in medical diagnostics is the development of an AI system that can detect breast cancer from mammography images with greater accuracy than human radiologists. The system, developed by researchers at Google Health, uses a CNN to analyze mammography images and identify abnormalities, such as tumors and calcifications. In a study published in the journal Nature, the system was found to be more accurate than human radiologists in detecting breast cancer.
2. Recurrent Neural Networks (RNNs): Analyzing Medical Time-Series Data
Recurrent Neural Networks (RNNs) are a type of AI algorithm that are particularly useful in analyzing medical time-series data, such as ECG signals, blood pressure readings, and glucose levels. RNNs are designed to analyze sequential data, identifying patterns and anomalies that may not be apparent to human clinicians.
One notable application of RNNs in medical diagnostics is the development of an AI system that can predict sepsis risk in patients. Sepsis is a life-threatening condition that occurs when the body's response to an infection becomes uncontrolled. The system, developed by researchers at the University of California, San Francisco, uses an RNN to analyze patient data, including vital signs, laboratory results, and medical history, to predict the risk of sepsis.
3. Natural Language Processing (NLP): Unlocking the Power of Medical Literature
Natural Language Processing (NLP) is a type of AI algorithm that enables computers to analyze and understand human language. In medical diagnostics, NLP has been used to unlock the power of medical literature, enabling clinicians to quickly identify relevant research studies, analyze scientific papers, and identify potential treatment options.
One notable example of the power of NLP in medical diagnostics is the development of an AI system that can analyze medical literature to identify potential treatments for rare diseases. The system, developed by researchers at the National Institutes of Health, uses NLP to analyze scientific papers, identifying potential treatments and identifying gaps in existing research.
4. Gradient Boosting Machines: Predicting Patient Outcomes
Gradient Boosting Machines are a type of AI algorithm that are particularly useful in predicting patient outcomes. These algorithms are designed to analyze large datasets, identifying complex patterns and relationships that may not be apparent to human clinicians.
One notable application of Gradient Boosting Machines in medical diagnostics is the development of an AI system that can predict patient outcomes following cardiac surgery. The system, developed by researchers at the University of California, Los Angeles, uses a Gradient Boosting Machine to analyze patient data, including medical history, laboratory results, and surgical data, to predict the risk of complications and mortality.
5. Random Forest Algorithms: Identifying High-Risk Patients
Random Forest Algorithms are a type of AI algorithm that are particularly useful in identifying high-risk patients. These algorithms are designed to analyze large datasets, identifying complex patterns and relationships that may not be apparent to human clinicians.
One notable application of Random Forest Algorithms in medical diagnostics is the development of an AI system that can identify high-risk patients with chronic kidney disease. The system, developed by researchers at the University of California, San Francisco, uses a Random Forest Algorithm to analyze patient data, including laboratory results, medical history, and demographic data, to identify patients at high risk of kidney failure.
6. Support Vector Machines (SVMs): Detecting Cancer Biomarkers
Support Vector Machines (SVMs) are a type of AI algorithm that are particularly useful in detecting cancer biomarkers. SVMs are designed to analyze high-dimensional data, identifying complex patterns and relationships that may not be apparent to human clinicians.
One notable application of SVMs in medical diagnostics is the development of an AI system that can detect cancer biomarkers in blood samples. The system, developed by researchers at the University of California, San Diego, uses an SVM to analyze blood samples, identifying biomarkers that may indicate the presence of cancer.
7. Autoencoders: Analyzing Medical Sensor Data
Autoencoders are a type of AI algorithm that are particularly useful in analyzing medical sensor data, such as EEG signals, ECG signals, and blood glucose levels. Autoencoders are designed to identify complex patterns and relationships in high-dimensional data, enabling clinicians to identify potential health risks and diagnose conditions more accurately.
One notable application of Autoencoders in medical diagnostics is the development of an AI system that can analyze EEG signals to diagnose neurological disorders, such as epilepsy and Parkinson's disease. The system, developed by researchers at the University of California, Los Angeles, uses an Autoencoder to analyze EEG signals, identifying patterns and anomalies that may not be apparent to human clinicians.
Conclusion
AI algorithms are revolutionizing medical diagnostics, enabling healthcare professionals to analyze vast amounts of medical data, identify patterns, and make accurate diagnoses with unprecedented speed and precision. From Convolutional Neural Networks to Autoencoders, these algorithms are transforming the way doctors diagnose and treat patients, improving patient outcomes, and reducing healthcare costs. As the field of medical diagnostics continues to evolve, it is likely that AI algorithms will play an increasingly important role, enabling clinicians to deliver more effective and personalized care to patients.