5 emerging trends in natural language processing
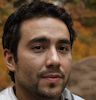

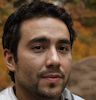
Unlocking the Power of Human Language: 5 Emerging Trends in Natural Language Processing
Natural Language Processing (NLP) has been a rapidly evolving field in recent years, with signifigant advancements in linguistic understanding, machine learning, and computational capabilities. As we move forward, NLP continues to transform the way humans interact with machines, and vice versa. In this article, we'll delve into the five emerging trends that are revolutionizing the NLP landscape, driving innovation, and shaping the future of human-machine communication.
Trend 1: Multimodal Language Processing
Traditional NLP has focused primarily on text-based inputs, but the rise of multimodal language processing is changing the game. This trend involves integrating various modalities, such as vision, speech, and gestures, to create more comprehensive and human-like understanding. Multimodal NLP enables machines to interpret and generate content that incorporates multiple senses, mimicking human communication.
For instance, Google's Multimodal Transformer model can analyze and generate content that combines text, images, and audio. This technology has far-reaching applications in areas like customer service, where chatbots can engage users through voice, text, and visual responses. Imagine a virtual assistant that can not only understand voice commands but also respond to gestures and facial expressions. This technology has the potential to revolutionize the way we interact with machines, making it more intuitive and human-like.
Trend 2: Explainable AI (XAI) in NLP
As NLP models become increasingly complex, it's essential to understand how they arrive at their conclusions. Explainable AI (XAI) in NLP aims to provide transparency into the decision-making process, making models more interpretable and trustworthy.
XAI techniques, such as Model Interpretability and Attention Visualization, allow developers to peek into the black box of neural networks, identifying biases and improving model performance. This trend has significant implications for high-stakes applications, like healthcare and finance, where understanding AI-driven decisions is crucial. For instance, in sentiment analysis, XAI can help developers understand why a model is classifying a particular text as positive or negative. This transparency is essential for building trust in AI systems and identifying potential biases.
Trend 3: Low-Resource Language Support
The overwhelming majority of NLP research focuses on high-resource languages like English, Chinese, and Spanish. However, this leaves millions of people speaking low-resource languages without access to NLP-powered tools and services.
Low-resource language support aims to bridge this gap by developing more efficient and adaptive models that can learn from limited data. Researchers are exploring techniques like transfer learning, meta-learning, and data augmentation to enable NLP capabilities for underrepresented languages. A recent study demonstrated the effectiveness of a multilingual model in processing African languages, highlighting the potential for NLP to support linguistic diversity and promote digital inclusivity.
Trend 4: Conversational AI with Emotional Intelligence
Conversational AI has made tremendous progress in recent years, but most chatbots still lack emotional intelligence. This trend seeks to infuse AI systems with empathy, understanding, and social awareness, enabling them to respond more appropriately to users' emotional cues.
Emotion-aware NLP models can analyze tone, sentiment, and context to provide more personalized and empathetic responses. This has significant implications for customer service, mental health support, and social companionship. For example, Woebot, a chatbot developed by Stanford University researchers, uses NLP and machine learning to detect and respond to users' emotional states, providing a more human-like interaction.
Trend 5: Adversarial NLP and Robustness
As NLP models become more pervasive, they also become attractive targets for malicious attacks. Adversarial NLP aims to develop models that can withstand such attacks, ensuring the robustness and security of NLP systems.
Researchers are exploring techniques like adversarial training, input preprocessing, and model ensembling to create more resilient models. This trend is critical for high-stakes applications, such as fraud detection, where NLP systems must withstand sophisticated attacks. A recent study demonstrated the vulnerability of popular NLP models to targeted attacks, highlighting the need for adversarial NLP to protect against potential threats.
Conclusion
The five emerging trends in NLP – multimodal language processing, XAI, low-resource language support, conversational AI with emotional intelligence, and adversarial NLP – are driving innovation and transforming the NLP landscape. As these trends continue to evolve, we can expect more sophisticated and human-like interactions between humans and machines.
The implications of these trends are far-reaching, with potential applications in areas like customer service, healthcare, education, and beyond. As NLP continues to advance, it's essential to prioritize transparency, accountability, and social responsibility, ensuring that these powerful technologies benefit humanity as a whole.
In conclusion, the future of NLP is bright, and it's exciting to think about the potential applications and implications of these emerging trends. As we unlock the power of human language, we can expect to see more intelligent, responsive, and empathetic machines that can interact with humans in a more natural and intuitive way.